Machine learning models for risk assessment of chemical compounds lack transparency, leading to skepticism among toxicologists and regulatory authorities. To address this, researchers at the University of Vienna developed the MolCompass software tool to identify areas where these models are weak. The RISK-HUNT3R project aims to develop non-animal risk assessment methods, supported by the European Union. Sergey Sosnin focused on binary classification models, highlighting the importance of confidence in predictions. The software aids in visual analysis of chemical space, allowing users to identify model blind spots. This approach aims to improve understanding of computational model limitations and reduce the need for animal testing in toxicology.
Source link
A breakthrough in identifying weak areas of chemical prediction models
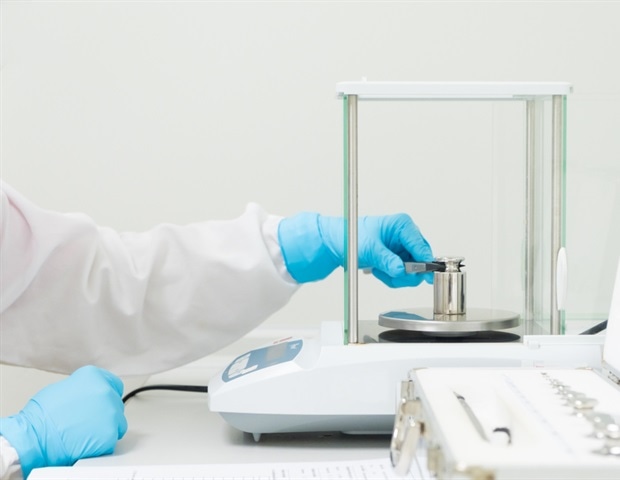